Publication 12 March 2025
Interactions and overlaps between the GDPR and AI Act, with Etienne Drouard
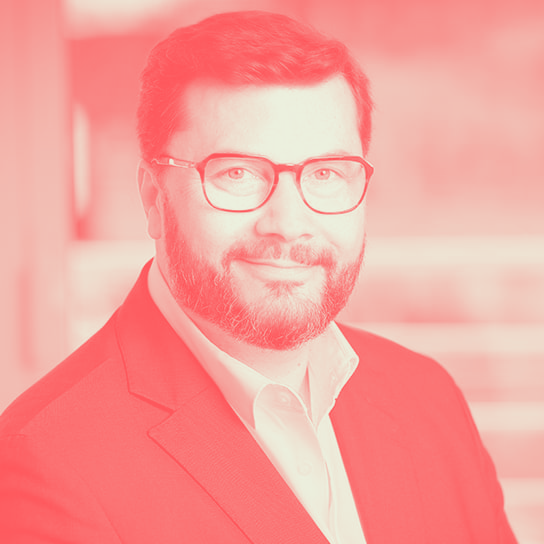
This interview is based on the article “The Interplay between the AI Act and the GDPR: Part I – When and How to Comply with Both”, published in the Journal of AI Law and Regulation, Volume 1 (2024), Issue 2, pp. 164 – 176, authored by Etienne Drouard, Olga Kurochkina, Rémy Schlich and Daghan Ozturk
What are the primary challenges in harmonising the AI Act and General Data Protection Regulation (GDPR)?
Harmonising the AI Act with the GDPR presents several challenges, as both aim to safeguard individual rights but through different lenses. The GDPR prioritises micro-level protections around personal data, ensuring that processing activities respect individual rights like privacy and consent. In contrast, the AI Act addresses broader societal issues, focusing on reducing systemic risks such as discrimination, bias, and unfair profiling by AI systems.
A significant challenge is the compatibility of these goals: the AI Act’s preventive risk categorisation clashes with GDPR’s accountability principle, where compliance is more individualised and requires operators to justify lawful grounds for processing personal data. Additionally, there’s an operational challenge in integrating different types of assessments — such as the data protection impact assessment (DPIA) under GDPR and the fundamental right impact assessment under the AI Act. These assessments can overlap, leading to duplicative or conflicting obligations for organisations developing or deploying AI systems.
Another concern is the legal ambiguity surrounding pseudonymisation and anonymisation in AI. Given AI’s iterative and data-intensive nature, defining when data is no longer personal or re-identifiable is complex. This uncertainty challenges compliance efforts and creates potential bottlenecks, especially where pseudonymised data might still indirectly identify individuals, thus falling under the scope of the GDPR. These frameworks will require enhanced collaboration between data protection and AI regulatory bodies to enable harmonisation.
Etienne Drouard
Partner, Hogan Lovells
How precisely do AI Act requirements regarding high-risk AI systems interact with GDPR’s impact assessments?
Like I just said, the AI Act and GDPR require different forms of risk assessments that often intersect, particularly for high-risk AI systems. Under the GDPR, organisations must perform data protection impact assessments (DPIAs) to assess data privacy risks, while the AI Act introduces fundamental rights impact assessments, specifically addressing risks of bias, discrimination, and other societal impacts of AI applications.
For high-risk AI systems, these assessments are designed to ensure that AI technologies are both lawful under the GDPR and socially responsible under the AI Act. DPIAs under the GDPR traditionally focus on privacy risks and data protection under different scenarios, such as assessing how a data processing operation could impact individual freedoms, especially in case of data breach. The AI Act broadens this focus to include a comprehensive biases assessment placing key societal rights issues at the center of the assessment, and mandating additional safeguards in cases where AI applications could harm fundamental rights or societal interests.
The challenge lies in creating an efficient process that harmonises both assessments without duplicative efforts. Some experts suggest a combined assessment model that fulfils both GDPR and AI Act requirements, although this might depend on cross-regulatory cooperation and streamlined guidelines. Ultimately, these assessments must align to promote trustworthy AI development that meets privacy and ethical standards in a unified framework.
How does the GDPR’s data minimisation principle apply to the development of AI systems, especially during the training phase?
The GDPR’s data minimisation principle mandates that only data necessary for a specific purpose be processed. Applying this principle in AI development, particularly in training phases, is complex due to the data-heavy requirements of training algorithms effectively. Large datasets help reduce bias and improve AI performance. Therefore, processing vast amounts of data seems, at first glance, to contradict the minimisation principle.
To address this, the GDPR does allow some flexibility in interpreting “necessity,” recognising that while vast datasets may be needed initially, data should be minimised post-collection. AI developers must thus implement robust filtering mechanisms that remove unnecessary data after the training process. This aligns with the CNIL’s guidance, which suggests that large datasets are permissible if developers anonymise or delete extraneous data post-processing.
The AI Act’s requirements further supplement GDPR principles by mandating representative, error-free, and comprehensive datasets for high-risk AI systems. In practice, both the GDPR and AI Act encourage a phased approach to data minimisation, wherein data is first collected broadly for training, then minimised through filtering, anonymisation, and deletion before deployment. Such a balanced approach ensures high-quality AI training while still respecting the GDPR’s core requirements.
Etienne Drouard
Partner, Hogan Lovells
Could you explain what is the ‘legitimate interests’ legal basis under the GDPR and its importance in AI systems development?
Under the GDPR, the “legitimate interest” basis is one of the lawful grounds for processing personal data, requiring a balancing test between the interests of the data controller and the rights of the data subject. In AI development, particularly during training phases, it’s impossible to collect free, specific, informed and unambiguous consent, and often impractical to establish a contractual basis with relevant data subjects for using personal data, making legitimate interest the primary legal basis.
Using legitimate interest requires developers to prove that processing personal data is necessary for their development goals and that this does not disproportionately infringe upon individuals’ rights. This balancing can be challenging, especially when AI training data involves indirectly collected personal data from third parties or public sources. Additionally, as AI systems may process vast amounts of data without immediate interaction with data subjects, fulfilling transparency and opt-out rights under GDPR remains extremely complex.
Despite these challenges, legitimate interest remains crucial in the AI context, as it provides flexibility when direct consent isn’t feasible. Regulators like the UK ICO are exploring this basis’s adaptability to AI, potentially relaxing strict interpretations to account for the unique demands of AI development. Still, without a harmonised approach across jurisdictions, relying on legitimate interest can introduce regulatory risk, underscoring the need for clear, industry-specific guidelines.
What implications do AI systems have for the GDPR’s ‘purpose limitation’ principle?
The GDPR’s purpose limitation principle requires that personal data collected for one specific purpose should not be repurposed in ways incompatible with its original intention. This presents a notable challenge for AI systems, where initial training data, often collected for diverse and unspecified uses, may be repurposed multiple times for evolving AI applications.
Etienne Drouard
Partner, Hogan Lovells
In AI contexts, distinguishing between initial purposes, such as data collection for training, and subsequent applications, such as data reuse for model improvement, can be challenging. The GDPR does allow for flexibility under compatibility criteria, yet determining if a secondary purpose aligns with the initial intent is less clear with AI’s iterative processes. For instance, if training data is later used for real-world decision-making applications, it raises concerns about whether the purpose remains “compatible.”
AI systems often operate with significant abstraction from the original data sources, further complicating compliance. Regulators have shown willingness to interpret purpose limitation pragmatically, acknowledging that AI’s evolving applications may necessitate broader initial purposes. However, more precise, case-by-case guidance is still needed to navigate purpose limitation effectively, particularly as AI development speeds ahead.
What recommendations would you give to policymakers and regulators to improve AI and GDPR alignment in the EU?
For a balanced regulatory environment that promotes innovation while protecting individual rights, policymakers should consider a few key recommendations. Firstly, enhancing regulatory clarity on the relationship between the AI Act and GDPR is critical. While the GDPR is designed for broad applicability, AI development has unique needs — such as large-scale data processing and continuous learning — that sometimes require interpretations beyond traditional data processing principles. Creating specific AI-focused guidance within the GDPR would help address this.
Another way to align both texts would be to introduce tailored compliance requirements for AI systems based on risk level, rather than applying uniform GDPR standards across all types of AI. For instance, high-risk AI applications in areas like healthcare or biometrics should rightly undergo stringent compliance, while low-risk AI tools could benefit from streamlined requirements. This tiered approach would maintain high standards without stifling innovation in lower-risk applications.
Etienne Drouard
Partner, Hogan Lovells
Standardising impact assessments across the GDPR and AI Act would also be helpful, combining data protection and fundamental rights considerations into a single, coherent assessment. Currently, organisations need to perform separate assessments. Harmonising these processes could make compliance simpler and reduce redundancy.
Policymakers should further promote collaboration among data protection and AI regulatory bodies, fostering a unified interpretation of key principles, such as data minimisation and purpose limitation, especially for dynamic AI applications. Ensuring these bodies work closely will also help organisations navigate potential jurisdictional overlaps or conflicting interpretations.
Finally, supporting innovation-friendly policies is essential. Policymakers should encourage research and development by creating safe “regulatory sandboxes” where AI can be developed and tested in controlled environments. These sandboxes would allow organisations to explore new AI applications with temporary regulatory flexibility, as long as there are strict safeguards and oversight. Through these combined efforts, we can better align AI governance with the GDPR, paving the way for trustworthy and ethically responsible AI in the EU.